What is Threat Hunting?
Threat Hunting is a technique to identify adversaries within an organization that go undetected by traditional security tools.
While a traditional, reactive approach to cyber security often involves automated alerts received and investigated by a security team, threat hunting takes a proactive approach to seek out potential threats and vulnerabilities before they escalate into full-blown security incidents. The benefits of hunting include identifying hidden threats, reducing the dwell time of attackers, and enhancing overall detection and response capabilities.
Threat Hunting Methodology
There are many different methodologies and frameworks for threat hunting, including the Pyramid of Pain, the Sqrrl Hunting Loop, and the MITRE ATT&CK Framework. While there is not one gold standard on how to conduct threat hunts, the typical process can be broken down into several key steps:
Planning and Hypothesis Creation: Define the scope and objective of the threat hunt. Identify potential targets and predict activity that might be taking place.
Data Collection: Refining data collection methods and gathering data from various sources, including logs, network traffic, and endpoint data.
Data Processing: Data that has been collected needs to be processed to generate information.
Data Analysis: Processed data can then be analyzed for anomalies, indicators of compromise (IoCs), or patterns of suspicious behavior.
Threat Identification: Based on the analysis, threat hunters may identify potential threats or security incidents.
Response: Taking action to mitigate or eradicate identified threats if any.
Documentation and Dissemination: It is important to record any findings or actions taken during the threat hunting process to serve as lessons learned for future reference. Additionally, any new threats or tactics, techniques, and procedures (TTPs) discovered may be shared with the cyber threat intelligence team or the wider community.
Building a Threat Hunting Program
For organizations looking to implement threat hunting as part of their cyber security program, they will need both a data collection source and human analysts as threat hunters.
Data collection and analysis may often be performed through existing security tools including SIEM systems, Network Traffic Analysis tools, endpoint agents, and system logs. On the human side, experienced threat hunters may be hired into an organization, or existing SOC analysts may be upskilled to perform threat hunts.
Leveraging AI security tools such as Darktrace can help to lower the bar in building a threat hunting program, both in analysis of the data and in assisting humans in their investigations.
Threat Hunting in Darktrace
To illustrate the benefits of leveraging Darktrace in threat hunting, we can walk through an example hunt following the key steps outlined above.
Planning and Hypothesis Creation
The initial hypothesis used in defining the scope of a threat hunt can come from several sources: threat intelligence feeds, the threat hunter’s own experience, or an anomaly detection that has been highlighted by Darktrace.
In this case, let’s imagine that this hunt is focused on a recent campaign by an Advanced Persistent Threat (APT). Threat intel has provided known file hashes, Command and Control (C2) IP addresses and domains, and MITRE techniques used by the attacker. The goal is to determine whether any indicators of this threat are present in the organization’s environment.
Data Collection and Data Processing
Darktrace can be deployed to cover an organization’s entire digital estate, including passive network traffic monitoring, cloud environments, and SaaS applications. Self-Learning AI is applied to the raw data to learn normal patterns of life for a specific environment and to highlight deviations from normal that might represent a threat. This data gives threat hunters a starting point in analyzing logs, meta-data, and anomaly detections.
Data Analysis
In the data analysis phase, threat hunters can use the Darktrace platform to search for the IoCs and TTPs identified during planning.
When searching for IoCs such as IP addresses or domain names, hunters can query the environment through the Omnisearch bar in the Darktrace Threat Visualizer. This search can provide a summary of all devices or users contacting a suspicious endpoint. From here the hunters can quickly pivot to identify surrounding activity from the source device.

Alternately, Darktrace Advanced Search can be used to search for these IoCs, but it also supports queries for file hashes or more advanced searches based on ports, protocols, data volumes, etc.

While searching for known suspicious domains and IP addresses is straightforward, the real strength of Darktrace lies in the ability to highlight deviations from a device’s ‘normal’ pattern of life. Darktrace has many built-in behavioral models designed to detect common adversary TTPs, all mapped to the MITRE ATT&CK Framework.
In the context of our threat hunt, we know that our target APT uses the Remote Desktop Protocol (RDP) to move laterally within a compromised network, specifically leveraging MITRE technique T1021.001. As each Darktrace model is mapped to MITRE, the threat hunter can search and find specific detection models that may be of interest, in this case the model ‘Anomalous Connection / Unusual Internal Remote Desktop’. From here they can view any devices that may have triggered this model, indicating possible attacker activity.
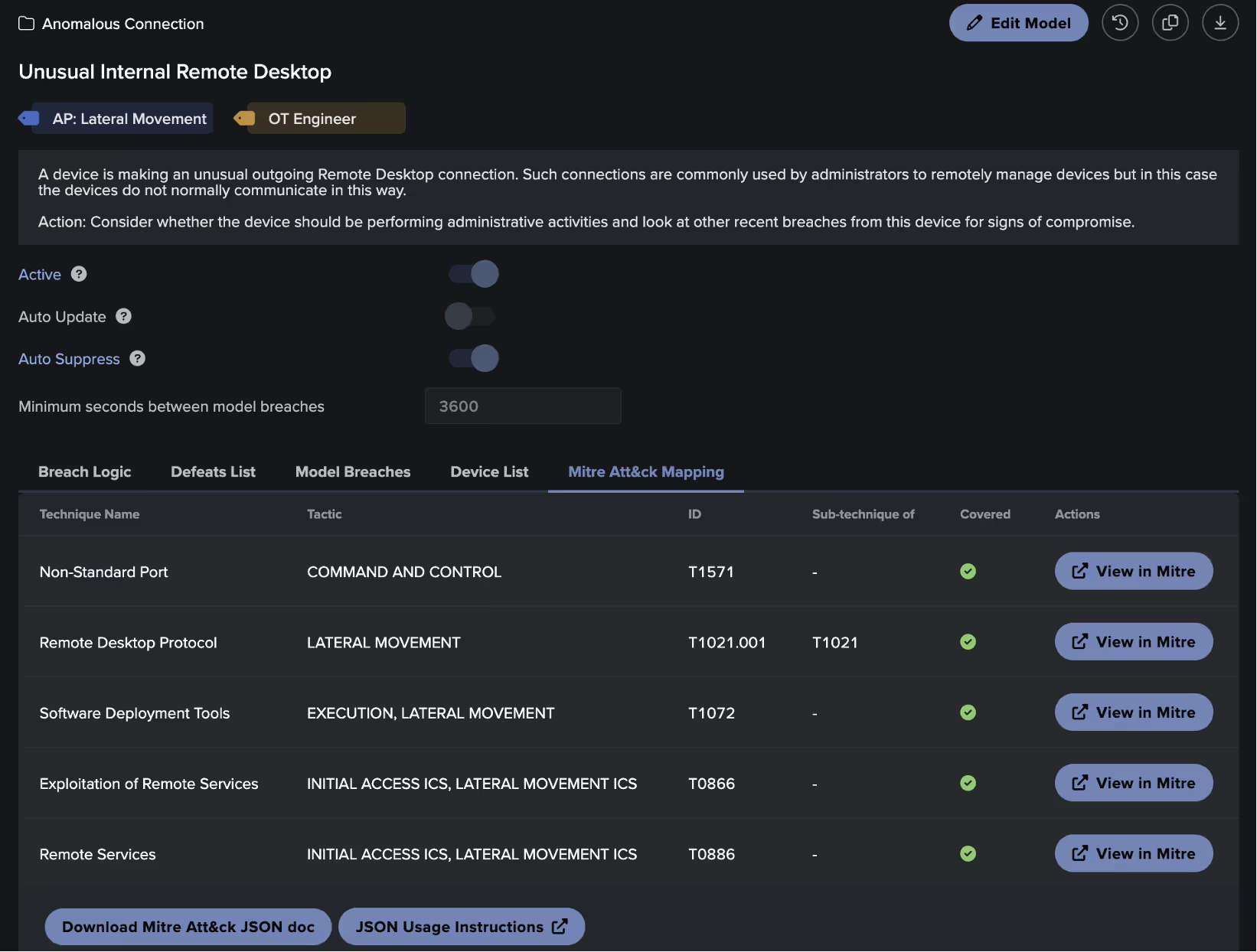
Threat hunters can also search more widely for any detections within a specific MITRE tactic through filters found on the Darktrace Threat Tray.
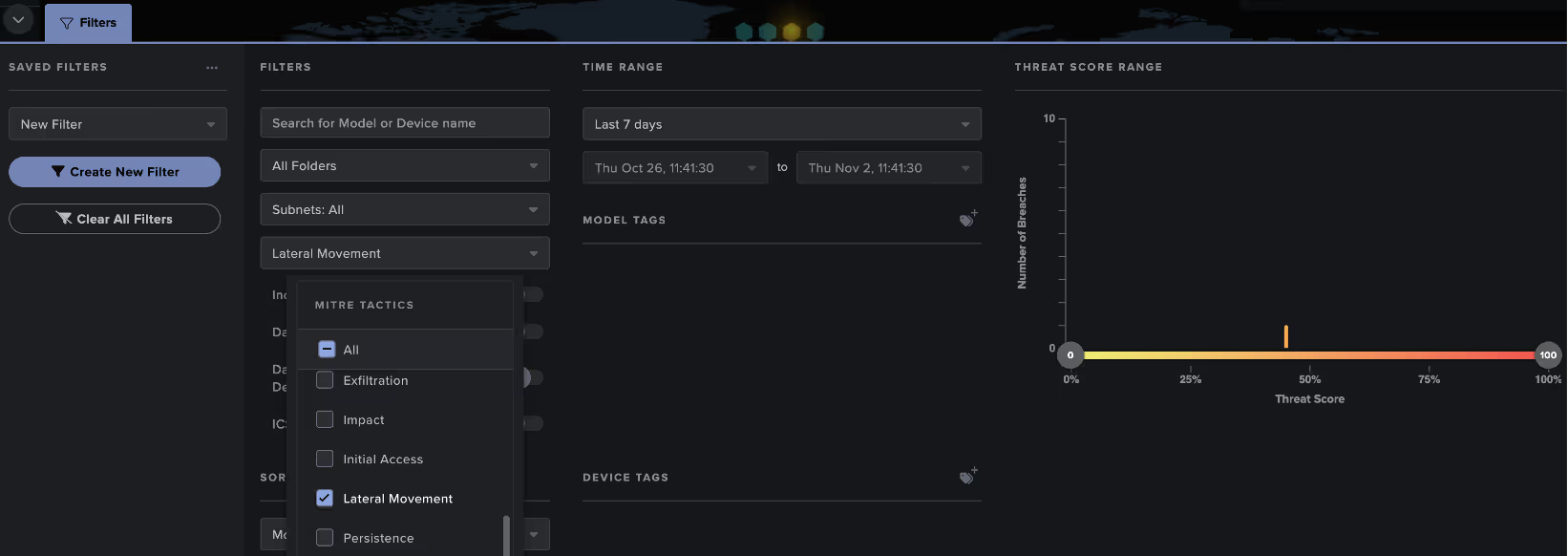
Threat Identification
Once a threat hunter has identified connections, model breaches, or anomalies during the analysis phase, they can begin to conduct further investigation to determine if this may represent a security incident.
Threat hunters can use Darktrace to perform deeper analysis through generating packet captures, visualizing surrounding network traffic, and utilizing features like the VirusTotal lookup to consult open-source intelligence (OSINT).
Another powerful tool to augment the hunter’s investigation is the Darktrace Cyber AI Analyst, which assists human teams in the investigation and correlation of behaviors to identify threats. Cyber AI Analyst automatically launches an initial triage of every model breach in the Darktrace platform, but threat hunters can also leverage manual investigations to gain additional context on their findings.
For example, say that an unusual RDP connection of interest was identified through Advanced Search. The hunter can pivot back to the Threat Visualizer and launch an AI Analyst investigation for the source device at the time of the connection. The resulting investigation may provide the hunter with additional suspicious behavior observed around that time, without the need for manual log analysis.
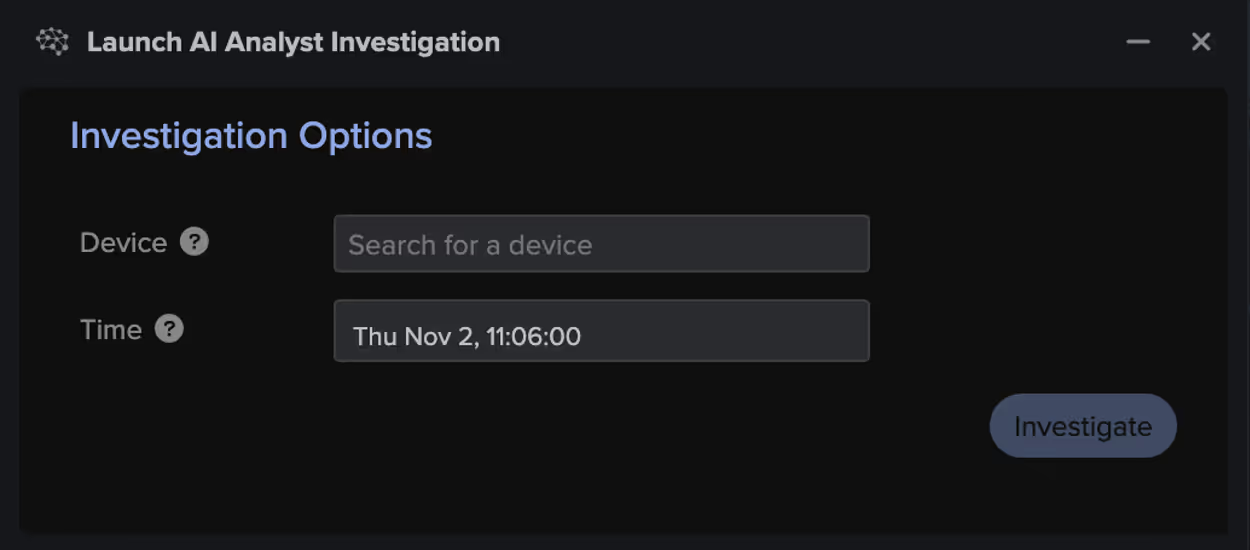
Response
If a threat is detected within Darktrace and confirmed by the threat hunter, Darktrace's Autonomous Response can be leveraged to take either autonomous or manual action to contain the threat. This provides the security team with additional time to conduct further investigation, pull forensics, and remediate the threat. This process can be further supported through the bespoke, AI-generated playbooks offered by Darktrace / Incident Readiness & Recovery, allowing an efficient recovery back to normal.
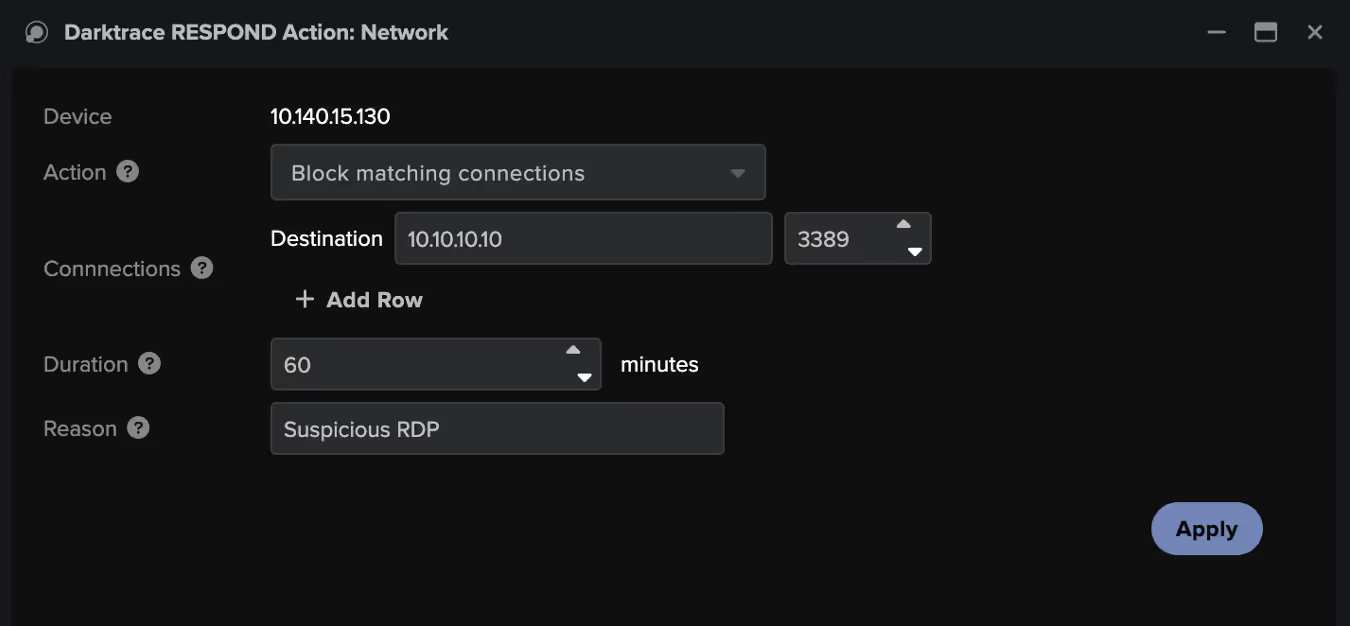
Documentation and Dissemination
An important final step is to document the threat hunting process and use the results to better improve automated security alerting and response. In Darktrace, reporting can be generated through the Cyber AI Analyst, Advanced Search exports, and model breach details to support documentation.
To improve existing alerting through Darktrace, this may mean creating a new detection model or increasing the priority of existing detections to ensure that these are escalated to the security team in the future. The Darktrace model editor provides users with full visibility into models and allows the creation of custom detections based on use cases or business requirements.

Conclusions
Proactive threat hunting is an important part of a cyber security approach to identify hidden threats, reduce dwell time, and improve incident response. Darktrace’s Self-Learning AI provides a powerful tool for identifying attacker TTPs and augmenting human threat hunters in their process. Utilizing the Darktrace platform, threat hunters can significantly reduce the time required to complete their hunts and mitigate identified threats.

Get the latest insights on emerging cyber threats
Attackers are adapting, are you ready? This report explores the latest trends shaping the cybersecurity landscape and what defenders need to know in 2025.
- Identity-based attacks: How attackers are bypassing traditional defenses
- Zero-day exploitation: The rise of previously unknown vulnerabilities
- AI-driven threats: How adversaries are leveraging AI to outmaneuver security controls
Stay ahead of evolving threats with expert analysis from Darktrace. Download the report here.