Ransomware, with more severe consequences and against increasingly high-stakes targets, continues to cause chaos and disruption to organizations globally. Earlier this year saw a surge in a strain of ransomware known as ‘Maze’, which shut down operations at leading optical products provider Canon and wreaked havoc in Fortune 500 companies like Cognizant.
Ransomware targeting healthcare
Just last month, news of a woman in Germany dying after a ransomware attack on the Dusseldorf University Hospital hit the headlines, confirming that the threat to people is no longer theoretical.
Ransomware affects all industries but 2020 has seen cyber-criminals increasingly hit essential services like healthcare, local government and critical infrastructure – intentionally or as collateral damage. As the stakes rise, so too does the need to understand how to prevent these devastating and pervasive attacks.
Once deployed, ransomware can spread laterally through an organization’s digital infrastructure in seconds, taking entire systems offline in minutes. Attackers often strike at night or at weekends, when they know security teams’ response time will be slower. Machine-speed attacks require machine-speed defenses that can detect and respond to this threat without human guidance, and autonomously block the threat.
This blog explains how AI detects and stops ransomware by learning ‘normal’ across the digital estate – from email and SaaS applications to the network, cloud, IoT and industrial control systems – by looking at an example of a Maze ransomware attack caught by Darktrace in a customer’s environment.
Darktrace’s Immune System detected the threat as soon as it emerged, but as the Autonomous Response capability was configured in passive mode, neutralizing the threat still required human action. This means that attackers were able to move laterally across the organization at speed and began to encrypt files before the security team stepped in. In active mode, Antigena Network would have contained the activity in its earliest stages.
How does Darktrace detect ransomware like Maze?
As soon as Darktrace is deployed – whether virtually or on-premise – the AI begins to learn the ‘pattern of life’ for every user and device across the organization. This enables the technology to detect anomalous activity indicative of a cyber-threat. It does this without relying on hard-coded rules and signatures; an approach that requires a ‘Patient Zero’ before updating these lists and containing subsequent identical threats. When it comes to a novel instance of ransomware spreading across an organization and infecting hundreds of devices in seconds, such an approach becomes useless.
With an understanding of the organization’s ‘pattern of life’, Darktrace’s AI recognizes unusual activity in real time. Such activity might include:
ActivityDarktrace detectionsUnusual downloads from C2 serversEXE from Rare Destination / Masqueraded File TransferBrute forcing publicly accessible RDP serversIncoming RDP brute force modelsBrute forcing access to web portal user accounts with weak passwords or lacking MFAVarious brute force modelsC2 via Cobalt Strike / Empire PowershellSSL Beaconing to Rare Endpoint / Empire Powershell and Cobalt Strike modelsNetwork scanning for reconnaissance & EternalBlue exploitSuspicious Network Scan model known to download Advanced IP Scanner after successful exploitMimikatz usage for privilege escalationUnusual Admin SMB Session / Unusual RDP Admin Session (Procdump, PingCastle, and Bloodhound)Psexec / ‘Living off the Land’ for lateral movementUnusual Remote Command Execution / Unusual PSexec / Unusual DCE RPCData exfiltration to C2 serversData Sent to Rare Domain / Unusual Internal Download / Unusual External UploadEncryptionSuspicious SMB Activity / Additional File Extensions AppendedExfiltration of passwords through various cloud storage servicesData Sent to New External DomainRDP tunnels using NgrokOutbound RDP / Various beaconing models
In addition, Darktrace is able to identify attempts to brute force access on Internet-facing servers. It can also detect specific searches for passwords stored in plain text as well as various password manager databases.
Maze ransomware analysis

Figure 1: A timeline of the attack
Most recently, Darktrace’s AI detected a case of Maze ransomware targeting a healthcare organization. Darktrace’s Immune System spotted every stage of the attack lifecycle within seconds, and the Cyber AI Analyst immediately launched an automated investigation of the full incident, surfacing a natural-language, actionable summary for the security team.
The initial infection vector was spear phishing. Maze is frequently delivered to healthcare organizations using pandemic-themed phishing emails. Darktrace also offers AI-powered email security that understands normal behavior for every Microsoft 365 user and spots anomalies that are indicative of phishing, but in the absence of this protection, the emails were waved through by traditional gateways.
The attacker began engaging in network scanning activity and enumeration to escalate access within the Research and Development subnet. Darktrace’s AI detected a successful compromise of admin level credentials, unusual RDP activities and multiple Kerberos authentication attempts.
Darktrace detected the attacker uploading a domain controller, before batch files were written to multiple file shares, which were used for the encryption process.
An infected device then connected to a suspicious domain that is associated to Maze mazedecrypt[.]top and the TOR browser bundle was downloaded, likely for C2 purposes. A large volume of sensitive data from the R&D subnet was then uploaded to a rare domain. This is typical of Maze ransomware, which is seen as a ‘double threat’ in that it not only seeks to encrypt critical files but also sends a copy of them back to the attacker.
This form of attack, also known as doxware, then provides the attacker with leverage in the possible event that the organization refused to pay the ransom – they can sell the data on the Dark Web, or threaten to leak intellectual property to competitors, for instance.
Real-time automated investigations with Cyber AI Analyst
Throughout the attack lifecycle, multiple high-fidelity alerts were generated by Darktrace AI and this prompted the Cyber AI Analyst to automatically launch an investigation in the background, stitching together the different events into a single, comprehensive security incident, which it then displayed for human review in a single screen.

Figure 2: The data exfiltration to a rare external domain
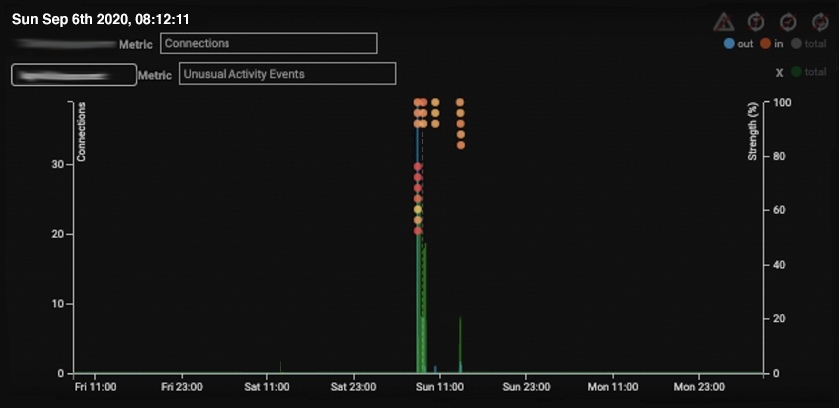
Figure 3: Darktrace’s user interface highlighting the unusual activity and model breaches on a domain controller directly linked with the ransomware attack
Targeted, double-threat attacks like Maze ransomware are on the rise and extremely dangerous – and they are increasingly targeting high-stakes environments. Thousands of organizations are turning to AI, not only to detect and investigate on ransomware intrusions as demonstrated above, but to autonomously respond to events as they occur. Ransomware attacks like these show organizations why autonomous response in active mode is not just a nice to have – but necessary – as fast-moving threats demand machine-speed responses.
In a previous blog, we looked at a novel zero-day ransomware attack that slipped through legacy security tools – but Antigena Network was configured in active mode, autonomously stopping the threat in its tracks. This unique capability is becoming crucial for organizations in every industry who find themselves targeted by increasingly sophisticated attack methods.
Thanks to Darktrace analyst Adam Stevens for his insights on the above threat find.
Learn more about Autonomous Response
Darktrace model detections
- Device / Suspicious Network Scan Activity
- Device / Network Scan
- Device / ICMP Address Scan
- Unusual Activity / Unusual Internal Connections
- Device / Multiple Lateral Movement Model Breaches
- Experimental / Executable Uploaded to DC
- Compromise / Ransomware::Suspicious SMB Activity
- Compromise / Ransomware::Ransom or Offensive Words Written to SMB
- Compliance / SMB Drive Write
- Compliance / High Priority Compliance Model Breach
- Anomalous Connection / SMB Enumeration
- Device / Suspicious File Writes to Multiple Hidden SMB Shares
- Device / New or Unusual Remote Command Execution
- Anomalous Connection / New or Uncommon Service Control
- Anomalous Connection / SMB Enumeration
- Experimental / Possible RPC Execution
- Anomalous Connection / High Volume of New or Uncommon Service Control
- Experimental / Possible Ransom Note
- Anomalous File / Internal::Additional Extension Appended to SMB File
- Compliance / Tor Package Download
- Device / Suspicious Domain
- Device / Long Agent Connection to New Endpoint
- Anomalous Connection / Data Sent to Rare Domain