Key takeaways
- A retail organization based in Africa was recently targeted with ransomware
- The general lack of obfuscation and use of no custom malware suggest a low-level threat actor
- Threat actors of all levels increasingly use common administrative tools such as PsExec for stealth purposes
- The company was relatively small, but no organization is immune to being targeted by ransomware
Attack details
Darktrace recently detected a form of ransomware at an African retailer. In the threat find that follows, the attacker connected to the organization’s domain controllers via a commonly used administrative tool and then began communicating to another C2 host.
Approximately an hour after the initial beaconing behavior, unusual RDP/SMB occurred on the network, followed by unusual service control activity. Darktrace detected each stage of the attack’s life cycle and would have automatically neutralized the attack had Darktrace Antigena been configured in active mode. However, because Autonomous Response was set up in passive mode, requiring confirmation from the human security team, the attack was able to escalate past its opening stages.
The ransomware activity commenced over the weekend, four days after the first beaconing activity. The timeline of the attack is shown below.
Timeline of attack: Overall dwell time around seven days
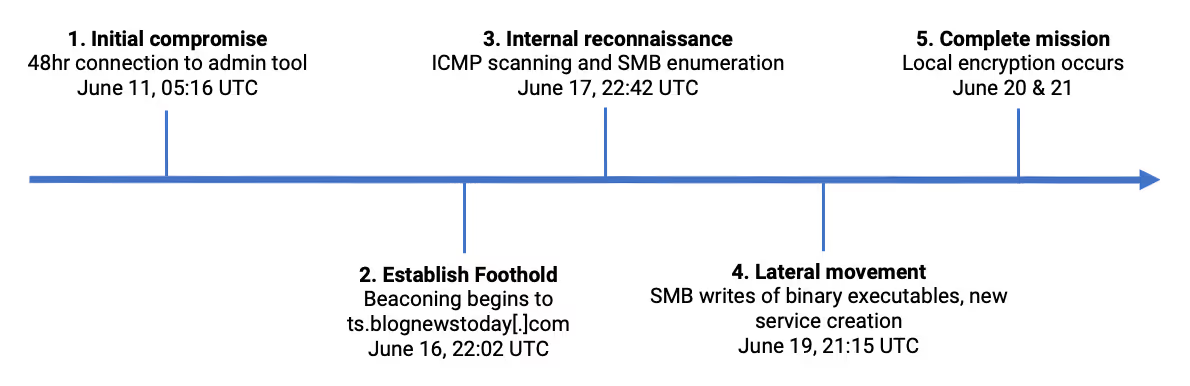
Figure 1: A timeline of events
How did the attack bypass the rest of the security stack?
This attack abused off-the-shelf tools that were already used by the client. This tactic, which targeted the domain controller as the initial vector, made the malware deployment easy and effective.
AI Analyst coverage
Darktrace’s Cyber AI analyst identified that the SQL server was writing a number of unusual files to shared drives, which appear to have specifically been binary executables for deployment of ransomware.

Figure 2: Darktrace’s Cyber AI Analyst revealing the unusual files
Overview of infected device
The graph below details the anomalous connections and other forms of unusual activity that occurred over a 10-hour period. Darktrace’s Enterprise Immune system first detected this activity in the compliance/remote management tool on the server, and then saw it spread laterally to other devices within the organization’s cyber-ecosystem.

Figure 3: A graph showing the number of external connections on the domain controller and anomalies detected
Concluding thoughts
In this attack, the C2 domain has an accessible array of standard PHP, including /phpMyAdmin and /p.php. The latter details the server time to be UTC+8, the time zone of mainland China.
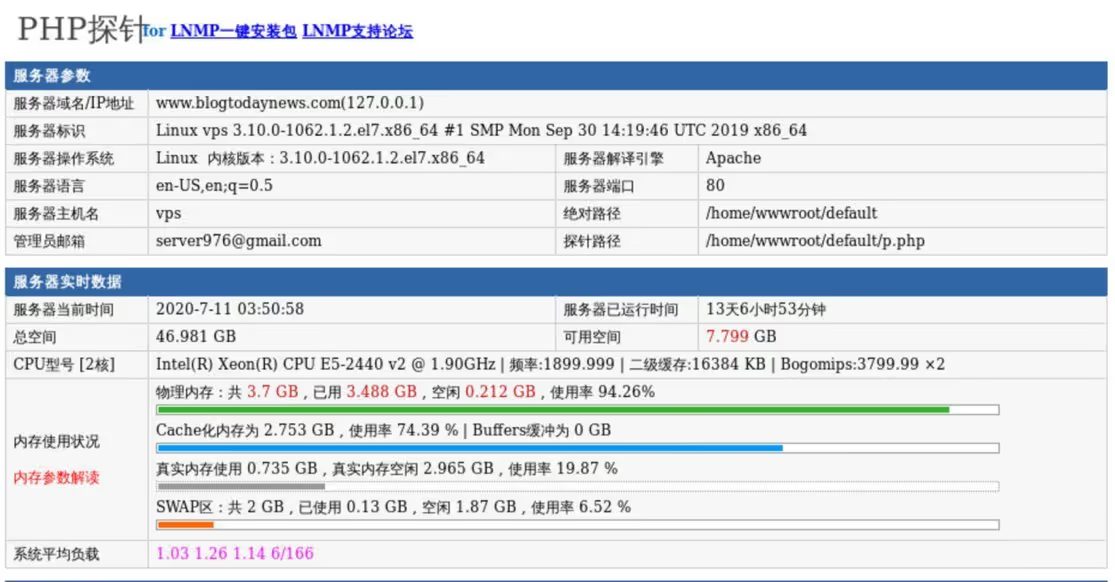
Figure 4: The C2 domain
Here, multiple factors suggest a lower-level threat actor, including the lack of obfuscation, the reliance on off-the-shelf tools, and the comparatively small size of the target organization. With the rise of Ransomware-as-a-Service (RaaS), automated domain generation, and other tools that lower the barrier to entry for attackers, it comes as no surprise that even a low-level threat actor could breach a corporate network. This also means that smaller organizations that would have been ignored by advanced cyber-criminals may find themselves targeted by attacks launched by low-level threat actors.
Indeed, convenient and widely used tools can often be abused for access, and the tools for ransomware are fairly common and easy to deploy once a foothold has been established. This calls for a proactive response to cyber security, and full visibility into networks, to be able to spot and stop threats before they escalate into crisis.
Deploying ransomware over the weekend is a common technique to maximize chances of success for the attacker, as response times from security teams are generally slower. This falls into a broader trend of ‘out of hours’ attacks that are becoming increasingly common and shines a light on the need for defensive technology that can act autonomously and contain a threat without relying on humans. With over a dozen AI models firing, there is no doubt that in this case Darktrace’s Autonomous Response technology would have taken a targeted and proportionate response to contain the threat. In addition to Autonomous Response, AI that can investigate an incident and provide actionable intelligence so a security team can quickly take action to fully remediate an incident or address a vulnerability is critical to staying ahead of fast-changing threats.
Thanks to Darktrace analyst Roberto Romeu for his insights on the above threat find.
Learn more about Autonomous Response
Darktrace model detections:
- Compromise / Suspicious Beaconing Behaviour
- Compromise / Sustained SSL or HTTP Increase
- Anomalous Server Activity / Rare External from Server
- Anomalous Server Activity / Anomalous External Activity from Critical Network Device
- Device / Network Scan
- Anomalous Connection / SMB Enumeration
- Device / ICMP Address Scan
- Device / New or Uncommon WMI Activity
- Anomalous Connection / New Service Control
- Anomalous Connection / New or Uncommon Service Control
- Anomalous Connection / Unusual Admin SMB Session
- Anomalous Connection / Active Remote Desktop Tunnel
- Anomalous Connection / Unusual Admin RDP Session
- Device / Multiple Lateral Movement Model Breaches
- Compliance / High Priority Compliance Model Breach
- Compliance / SMB Drive Write
- Compliance / Remote Management Tool On Server