One of the most enjoyable discussions (and debates) I engage in is the topic of Security Operations Center (SOC) and Incident Response (IR) metrics to measure and validate an organization’s Return on Investment (ROI). The debate part comes in when I hear vendor experts talking about “the only” SOC metrics that matter, and only list the two most well-known, while completely ignoring metrics that have a direct causal relationship.
In this blog, I will discuss what I believe are the SOC/IR metrics that matter, how each one has a direct impact on the others, and why organizations should ensure they are working towards the goal of why these metrics are measured in the first place: Reduction of Risk and Costs.
Reduction of Risk and Costs
Every security solution and process an organization puts in place should reduce the organization’s risk of a breach, exposure by an insider threat, or loss of productivity. How an organization realizes net benefits can be in several ways:
- Improved efficiencies can result in SOC/IR staff focusing on other areas such as advanced threat hunting rather than churning through alerts on their security consoles. It may also help organizations dealing with the lack of skilled security staff by using Artificial Intelligence (AI) and automated processes.
- A well-oiled SOC/IR team that has greatly reduced or even eliminated mundane tasks attracts, motivates, and retains talent resulting in reduced hiring and training costs.
- The direct impact of a breach such as a ransomware attack can be devastating. According to the 2024 Data Breach Investigations Report by Verizon, MGM Resorts International reported the ALPHV ransomware cost the company approximately $100 million[1].
- Failure to take appropriate steps to protect the organization can result in regulatory fines; and if an organization has, or is considering, purchasing Cyber Insurance, can result in declined coverage or increased premiums.
How does an organization demonstrate they are taking proactive measures to prevent breaches? That is where it's important to understand the nine (yes, nine) key metrics, and how each one directly influences the others, play their roles.
Metrics in the Incident Response Timeline
Let’s start with a review of the key steps in the Incident Response Timeline:
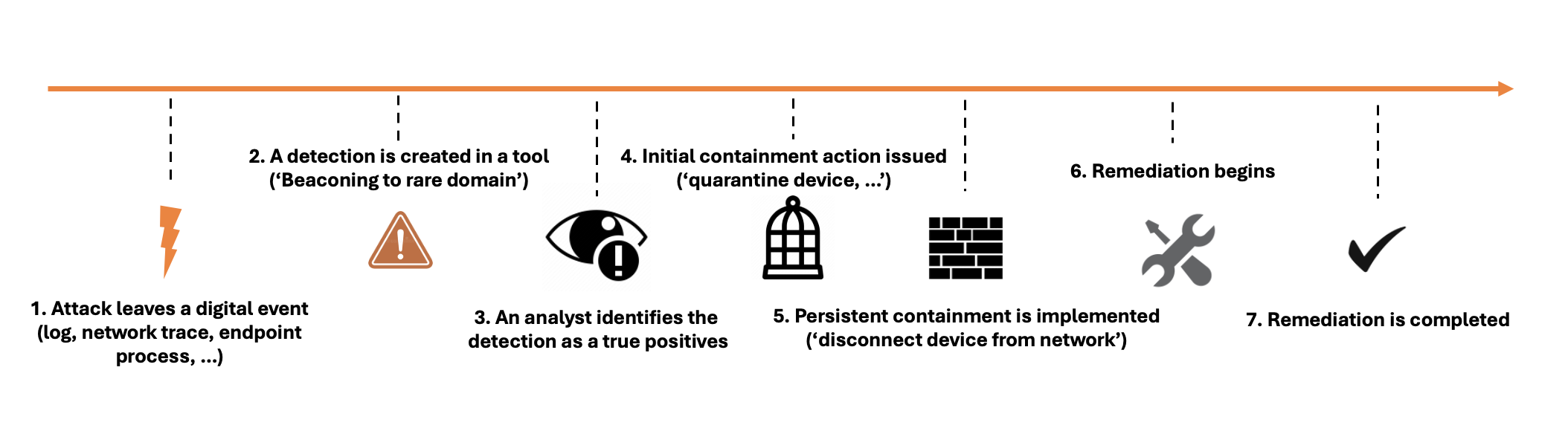
Seven of the nine key metrics are in the IR timeline, while two of the metrics occur before you ever have an incident. They occur in the Pre-Detection Stage.
Pre-Detection stage metrics are:
- Preventions Per Intrusion Attempt (PPIA)
- False Positive Reduction Rate (FPRR)
Next is the Detect and Investigate stage, there are three metrics to consider:
- Mean Time to Detection (MTTD)
- Mean Time to Triage (MTTT)
- Mean Time to Understanding (MTTU)
This is followed by the Remediation stage, there are two metrics here:
- Mean Time to Containment (MTTC)
- Mean Time to Remediation / Recovery (MTTR)
Finally, there is the Risk Reduction stage, there are two metrics:
- Mean Time to Advice (MTTA)
- Mean Time to Implementation (MTTI)
Pre-Detection Stage
Preventions Per Intrusion Attempt
PPIA is defined as stopping any intrusion attempt at the earliest possible stage. Your network Intrusion Prevention System (IPS) blocks vulnerability exploits, your e-mail security solution intercepts and removes messages with malicious attachments or links, your egress firewall blocks unauthorized login attempts, etc. The adversary doesn’t get beyond Step 1 in the attack life cycle.
This metric is the first domino. Every organization should strive to improve on this metric every day. Why? For every intrusion attempt you stop right out of the gate, you eliminate the actions for every other metric. There is no incident to detect, triage, investigate, remediate, or analyze post-incident for ways to improve your security posture.
When I think about PPIA, I always remember back to a discussion with a former mentor, Tim Crothers, who discussed the benefits of focusing on Prevention Failure Detection.
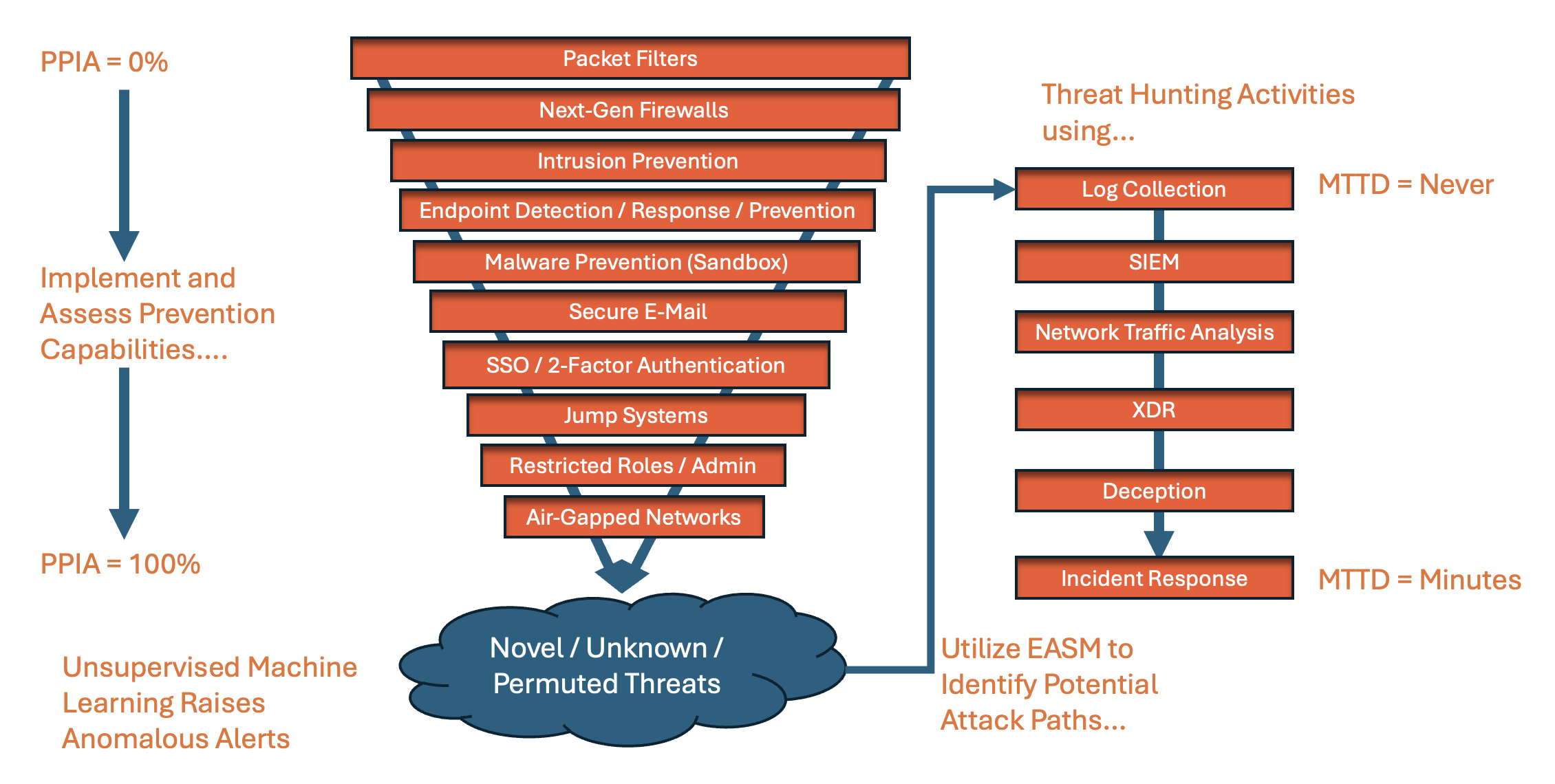
The concept is that as you layer your security defenses, your PPIA moves ever closer to 100% (no one has ever reached 100%). This narrows the field of fire for adversaries to breach into your organization. This is where novel, unknown, and permuted threats live and breathe. This is where solutions utilizing Unsupervised Machine Learning excel in raising anomalous alerts – indications of potential compromise involving one of these threats. Unsupervised ML also raises alerts on anomalous activity generated by known threats and can raise detections before many signature-based solutions. Most organizations struggle to find strong permutations of known threats, insider threats, supply chain attacks, attacks utilizing n-day and 0-day exploits. Moving PPIA ever closer to 100% also frees your team up for conducting threat hunting activities – utilizing components of your SOC that collect and store telemetry to query for potential compromises based on hypothesis the team raises. It also significantly reduces the alerts your team must triage and investigate – solving many of the issues outlined at the start of this paper.
False Positive Reduction Rate
Before we discuss FPRR, I should clarify how I define False Positives (FPs). Many define FPs as an alert that is in error (i.e.: your EDR alerts on malware that turns out to be AV signature files). While that is a FP, I extend the definition to include any alert that did not require triage / investigation and distracts the SOC/IR team (meaning they conducted some level of triage / investigation).
This metric is the second domino. Why is this metric important? Every alert your team exerts time and effort on that is a non-issue distracts them from alerts that matter. One of the major issues that has resonated in the security industry for decades is that SOCs are inundated with alerts and cannot clear the backlog. When it comes to PPIA + FPRR, I have seen analysts spend time investigating alerts that were blocked out of the gate while their screen continued to fill up with more. You must focus on Prevention Failure Detection to get ahead of the backlog.
Detect and Investigate Stages
Mean Time to Detection
MTTD, or “Dwell Time”, has decreased dramatically over the past 12 years. From well over a year to 16 days in 2023[2]. MTTD is measured from the earliest possible point you could detect the intrusion to the moment you actually detect it.
This third domino is important because the longer an adversary remains undetected, the more the odds increase they will complete their mission objective. It also makes the tasks of triage and investigation more difficult as analysts must piece together more activity and adversaries may be erasing evidence along the way – or your storage retention does not cover the breach timeline.
Many solutions focusing solely on MTTD can actually create the very problem SOCs are looking to solve. That is, they generate so much alerting that they flood the console, email, or text messaging app causing an unmanageable queue of alerts (this is the problem XDR solutions were designed to resolve by focusing on incidents rather than alerts).
Mean Time to Triage
MTTT involves SOCs that utilize Level 1 (aka Triage) analysts to render an “escalate / do not escalate” alert verdict accurately. Accuracy is important because Triage Analysts typically are staff new to cyber security (recent grad / certification) and may over escalate (afraid to miss something important) or under escalate (not recognize signs of a successful breach). Because of this, a small MTTT does not always equate to successful handling of incidents.
This metric is important because keeping your senior staff focused on progressing incidents in a timely manner (and not expending time on false positives) should reduce stress and required headcount.
Mean Time to Understanding
MTTU deals with understanding the complete nature of the incident being investigated. This is different than MTTT which only deals with whether the issue merits escalation to senior analysts. It is then up to the senior analysts to determine the scope of the incident, and if you are a follower of my UPSET Investigation Framework, you know understanding the full scope involves:
U = All compromised accounts
P = Persistence Mechanisms used
S = All systems involved (organization, adversary, and intermediaries)
E = Endgame (or mission objective)
T = Techniques, Tactics, Procedures (TTPs) utilized by the adversary
MTTU is important because this information is critical before any containment or remediation actions are taken. Leave a stone unturned, and you alert the adversary that you are onto them and possibly fail to close an avenue of access.
Remediation Stages
Mean Time to Containment
MTTC deals with neutralizing the threat. You may not have kicked the adversary out, but you have halted their progress to their mission objective and ability to inflict further damage. This may be through use of isolation capabilities, termination of malicious processes, or firewall blocks.
MTTC is important, especially with ransomware attacks where every second counts. Faster containment responses can result in reduced / eliminated disruption to business operations or loss of data.
Mean Time to Remediation / Recovery
The full scope of the incident is understood, the adversary has been halted in their tracks, no malicious processes are running on any systems in your organization. Now is the time to put things back to right. MTTR deals with the time involved in restoring business operations to pre-incident stage. It means all remnants of changes made by the adversary (persistence, account alterations, programs installed, etc.) are removed; all disrupted systems are restored to operations (i.e.: ransomware encrypted systems are recovered from backups / snapshots), compromised user accounts are reset, etc.
MTTR is important because it informs senior management of how fast the organization can recover from an incident. Disaster Recovery and Business Continuity plans play a major role in improving this score.
Risk Reduction Stages
Mean Time to Advice
After the dust has settled from the incident, the job is not done. MTTA deals with identifying and assessing the specific areas (vulnerabilities, misconfigurations, lack of security controls) that permitted the adversary to advance to the point where detection occurred (and any actions beyond). The SOC and IR teams should then compile a list of recommendations to present to management to improve the security posture of the organization so the same attack path cannot be used.
Mean Time to Implement
Once recommendations are delivered to management, how long does it take to implement them? MTTI tracks this timeline because none of it matters if you don’t fix the holes that led to the breach.
Nine Dominos
There are the nine dominos of SOC / IR metrics I recommend helping organizations know if they are on the right track to reduce risk, costs and improve morale / retention of the security teams. You may not wish to track all nine, but understanding how each metric impacts the others can provide visibility into why you are not seeing expected improvements when you implement a new security solution or change processes.
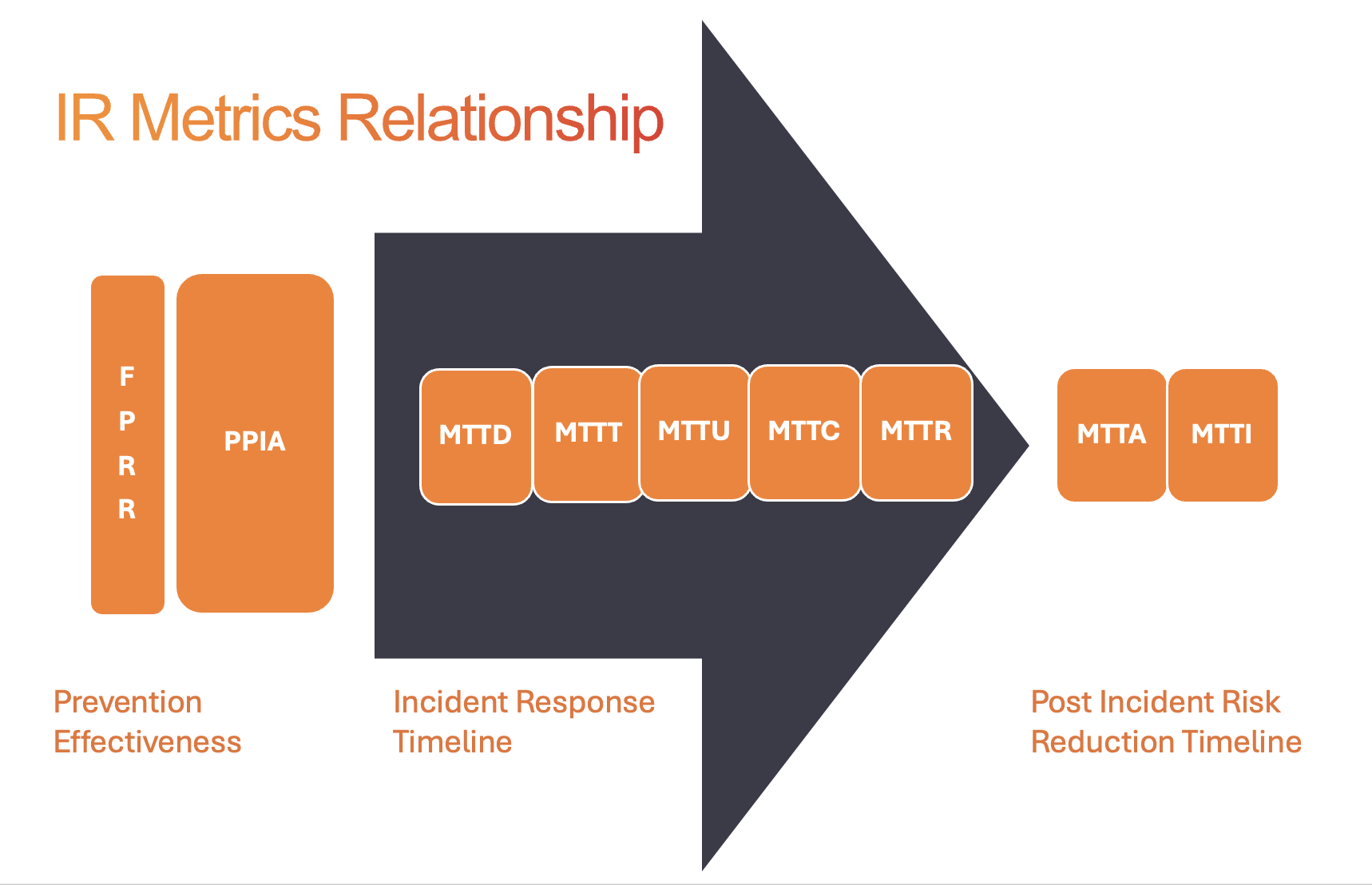
Improving prevention and reducing false positives can make huge positive impacts on your incident response timeline. Utilizing solutions that get you to resolution quicker allows the team to focus on recommendations and risk reduction strategies.
Whichever metrics you choose to track, just be sure the dominos fall in your favor.
References
[1] 2024 Verizon Data Breach Investigations Report, p83
[2] Mandiant M-Trends 2023