Recently, Darktrace ran a customer trial of our email security product for a leading European infrastructure operator looking to upgrade its email protection.
During this prospective customer trial, Darktrace encountered several security incidents that penetrated existing security layers. Two of these incidents were Business Email Compromise (BEC) attacks, which we’re going to take a closer look at here.
Darktrace was deployed for a trial at the same time as two other email security vendors, who were also being evaluated by the prospective customer. Darktrace’s superior detection of threats in this trial laid the groundwork for the respective company to choose our product.
Let’s dig into some of the elements of this Darktrace tech win and how they came to light during this trial.
Why truly intelligent AI starts learning from scratch
Darktrace’s detection capabilities are powered by true unsupervised machine learning, which detects anomalous activity from its ever-evolving understanding of normal for every unique environment. Consequently, it learns every business from the beginning, training on an organization’s data to understand normal for its users, devices, assets and the millions of connections between them.
This learning period takes around a week, during which the AI hones its understanding of the business to a precise degree. At this stage, the system may produce some noise or lack precision, but this is a testament to our unsupervised machine learning. Unlike solutions that promise faster results by relying on preset assumptions, our AI takes the necessary time to learn from scratch, ensuring a deeper understanding and increasingly accurate detection over time.
Real threats detected by Darktrace
Attack 1: Supply chain attack
BEC and supply chain attacks are notoriously difficult to detect, as they take advantage of established, trusted senders.
This attack came from a legitimate server via a known supplier with which the prospective customer had active and ongoing communication. Using the compromised account, the attacker didn’t just send out randomized spam, they crafted four sophisticated social engineering emails with the aim of soliciting users to click on a link – directly tapping into existing conversations. Darktrace / EMAIL was configured in passive mode during this trial; it would otherwise have held the emails before they arrived in the inbox. Luckily in this instance, one user reported the email to the CISO before any other users clicked the link. Upon investigation, the link contained timed ransomware detonation.
Darktrace was the only vendor that caught any of these four emails. Our unique behavioral AI approach enables Darktrace / EMAIL to protect customers from even the most sophisticated attacks that abuse prior trust and relationships.
How did Darktrace catch this attack that other vendors missed?
With traditional email security, security teams have been obliged to allow entire organizations to eliminate false positives – on the premise that it’s easier to make a broad decision based on an entire known domain and assume that potential risk of a supply chain attack.
By contrast, Darktrace adopts a zero trust mentality, analyzing every email to understand whether communication that has previously been safe remains safe. That’s why Darktrace is uniquely positioned to detect BEC, based on its deep learning of internal and external users. Because it creates individual profiles for every account, group and business composed of multiple signals, it can detect deviations in their communication patterns based on the context and content of each message. We think of this as the ‘self-learning’ vs ‘learning the breach’ differentiator.

If set in autonomous mode where it can apply actions, Darktrace / EMAIL would have quarantined all four emails. Using machine learning indicators such as ‘Inducement Shift’ and ‘General Behavioral Anomaly’, it deemed the four emails ‘Out of Character’. It also identified the link as highly likely to be phishing, based purely on its context. These indicators are critical because the link itself belonged to a widely used legitimate domain, leveraging their established internet reputation to appear safe.
Around an hour later the supplier regained control of the account and sent a legitimate email alerting a wide distribution list to the phishing emails sent. Darktrace was able to discern the previously sent malicious emails from the current legitimate emails and allowed these emails through. Compared to other vendors that have a static understanding of malicious which needs to be updated (in cases like this, once a supplier is de-compromised), Darktrace’s deep understanding of external entities enables further nuance and precision in determining good from bad.

Attack 2: Microsoft 365 account takeover
As part of building behavioral profiles of every email user, Darktrace analyzes their wider account activity. Account activity, such as unusual login patterns and administrative activity, is a key variable to detect account compromise before malicious activity occurs, but it also feeds into Darktrace’s understanding of which emails should belong in every user’s inbox.
When the customer experienced an account compromise on day two of the trial, Darktrace began an investigation and was able to provide the full breakdown and scope of the incident.
The account was compromised via an email, which Darktrace would have blocked if it had been deployed autonomously at the time. Once the account had been compromised, detection details included:
- Unusual Login and Account Update
- Multiple Unusual External Sources for SaaS Credential
- Unusual Activity Block
- Login From Rare Endpoint While User is Active
.avif)
With Darktrace / EMAIL, every user is analyzed for behavioral signals including authentication and configuration activity. Here the unusual login, credential input and rare endpoint were all clear signals a compromised account, contextualized against what is normal for that employee. Because Darktrace isn’t looking at email security merely from the perspective of the inbox. It constantly reevaluates the identity of each individual, group and organization (as defined by their behavioral signals), to determine precisely what belongs in the inbox and what doesn’t.
In this instance, Darktrace / EMAIL would have blocked the incident were it not deployed in passive mode. In the initial intrusion it would have blocked the compromising email. And once the account was compromised, it would have taken direct blocking actions on the account based on the anomalous activity it detected, providing an extra layer of defense beyond the inbox.
Account takeover protection is always part of Darktrace / EMAIL, which can be extended to fully cover Microsoft 365 SaaS with Darktrace / IDENTITY. By bringing SaaS activity into scope, security teams also benefit from an extended set of use cases including compliance and resource management.
Why this customer committed to Darktrace / EMAIL
“Darktrace was the only AI vendor that showed learning,” – CISO, Trial Customer
Throughout this trial, Darktrace evolved its understanding of the trial customer’s business and its email users. It identified attacks that other vendors did not, while allowing safe emails through. Furthermore, the CISO explicitly cited Darktrace as the only technology that demonstrated autonomous learning. As well as catching threats that other vendors did not, the CISO saw maturity areas such as how Darktrace dealt with non-productive mail and business-as-usual emails, without any user input. Because of the nature of unsupervised ML, Darktrace’s learning of right and wrong will never be static or complete – it will continue to revise its understanding and adapt to the changing business and communications landscape.
This case study highlights a key tenet of Darktrace’s philosophy – that a rules and tuning-based approach will always be one step behind. Delivering benign emails while holding back malicious emails from the same domain demonstrates that safety is not defined in a straight line, or by historical precedent. Only by analyzing every email in-depth for its content and context can you guarantee that it belongs.
While other solutions are making efforts to improve a static approach with AI, Darktrace’s AI remains truly unsupervised so it is dynamic enough to catch the most agile and evolving threats. This is what allows us to protect our customers by plugging a vital gap in their security stack that ensures they can meet the challenges of tomorrow's email attacks.
Interested in learning more about Darktrace / EMAIL? Check out our product hub.
Download: Darktrace / EMAIL Solution Brief
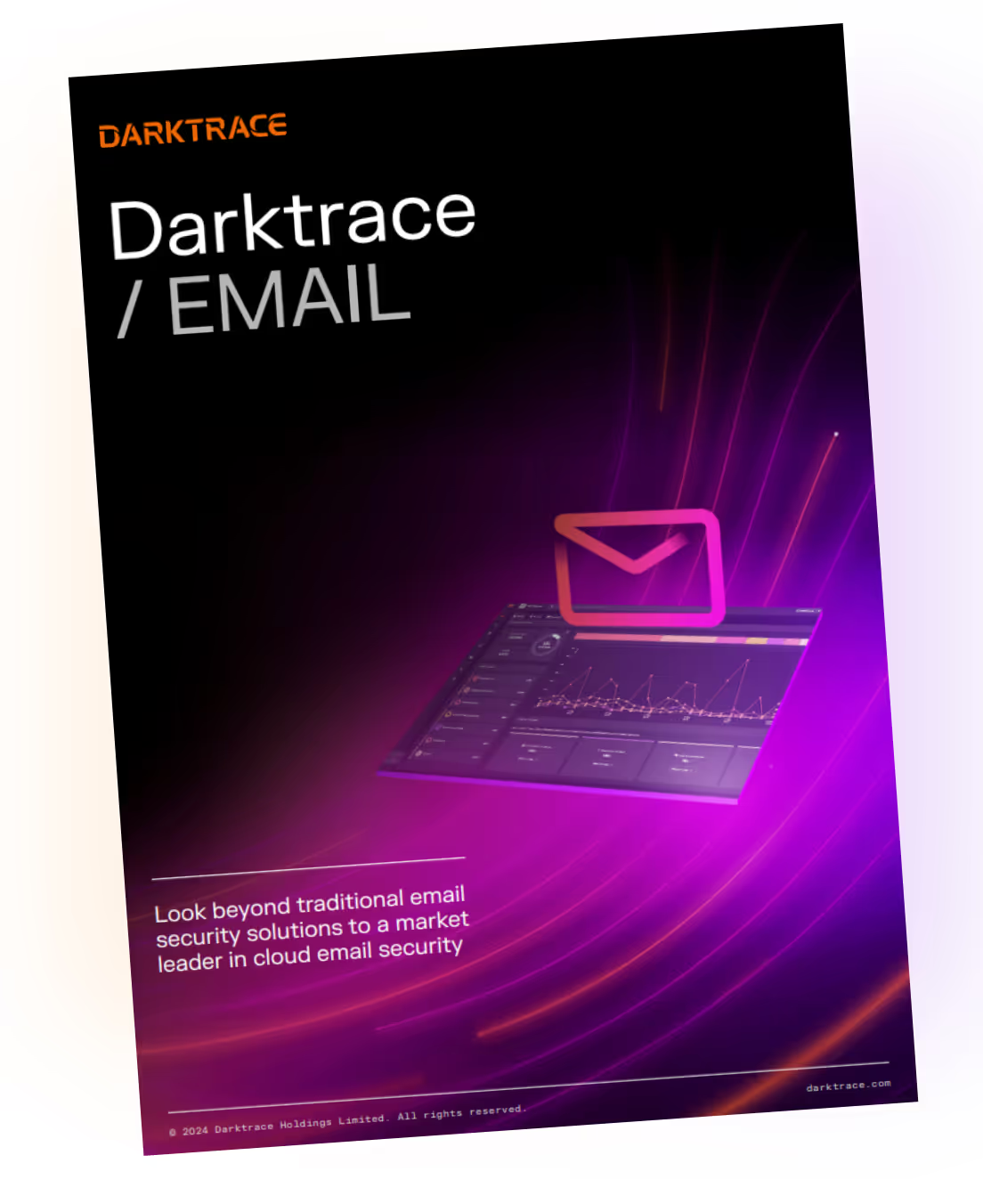
Discover the most advanced cloud-native AI email security solution to protect your domain and brand while preventing phishing, novel social engineering, business email compromise, account takeover, and data loss.
- Gain up to 13 days of earlier threat detection and maximize ROI on your current email security
- Experience 20-25% more threat blocking power with Darktrace / EMAIL
- Stop the 58% of threats bypassing traditional email security