Originally created by famed French programmer Benjamin Delpy to highlight security flaws in Windows authentication mechanisms, today Mimikatz is a staple post-exploitation module in the arsenal of cyber-criminals, since it facilitates lateral movement across a victim’s network. Mimikatz was a primary feature of the global ransomware attacks NotPetya and BadRabbit, in addition to the alleged Russian hacking of the German parliament in 2015 and 2017.
Among the primary vulnerabilities that Mimikatz exploits is Windows’ Local Security Authority Subsystem Service (LSASS), which is designed to obviate the need for users to reauthenticate every time they seek to access internal resources. Despite its clear utility, LSASS works by keeping a cache of every credential used since the last boot, presenting an obvious security risk in the event the cache is compromised. Broadly speaking, Mimikatz plunders this resource and allows users to access cleartext passwords as well as NTLM hashes. With this data in hand, threat actors are able to conduct the following attacks:
- Kerberos Golden Ticket: Provides administrative credentials for the whole domain.
- Pass-the-Ticket: Enables a user to pass a Kerberos ticket to a second device and login using this ticket.
- Kerberos Silver Ticket: Provides a TGS ticket to log into any network service.
- Pass-the-Hash: Allows a user to pass a hash string in order to login.
Dumping LSASS memory is just one method that Mimikatz and its many updated versions employ to harvest credentials. Indeed, once malware such as NotPetya has established itself on single device, the Mimikatz module can exploit a variety of security flaws to obtain the password information for any other users or computers that have logged onto that machine: a key step for both lateral movement and privilege escalation. Like many successful hacker tools, Mimikatz has inspired the creation of other programs with similar aims, which are largely intended to circumvent antivirus controls.
Using AI to end the cat-and-mouse game
At the most basic level, security teams can reduce vulnerability to Mimikatz and to lateral movement more generally by ensuring that each user has the minimum amount of privileges needed for his or her role. But while this measure is certainly prudent, it will not always be effective — especially when dealing with sophisticated threats. Another strategy is to implement endpoint security tools and anti-virus software, which rely on rules and signatures to detect known Mimikatz variants. However, as Mimikatz and its copycats continue to evolve, these traditional tools are locked in a ceaseless cat-and-mouse game, unable to spot unknown variants of Mimikatz that are designed to circumvent them.
As a fundamentally different approach to security, artificially intelligent systems like Darktrace avoid this cat-and-mouse game by learning what constitutes normal behavior for the users and devices they safeguard while “on the job,” rather than using fixed rules and signatures. This approach alerts defenders to any anomalous activity, regardless of whether such activity constitutes a known or unknown threat. Typically, lateral movement involving Mimikatz will involve a spike in unusual SMB activity, as attackers seek to write the tool to target devices. The following recent attack on a Darktrace customer highlights how AI can allow security teams to quickly detect and respond to such lateral movement involving Mimikatz:
- Darktrace alerts to a non-domain joined Linux device appearing on the customer’s network and engaging in extensive SMB bruteforce activity against key servers.
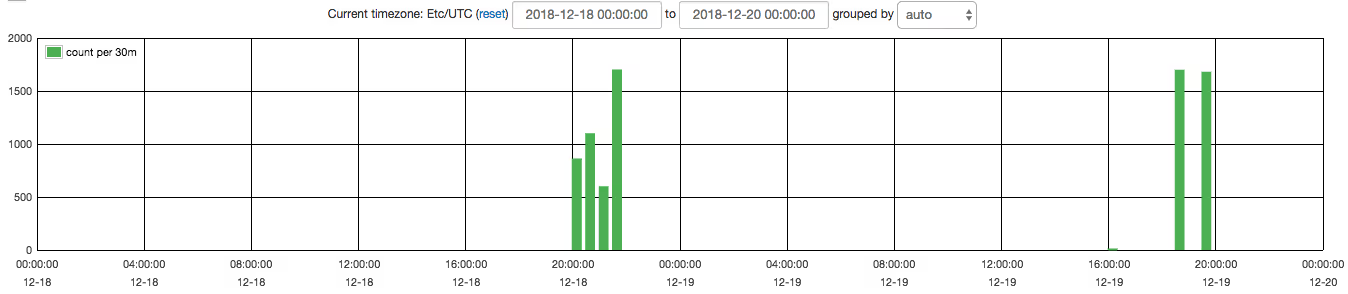
- Figure 1: Darktrace detects the spike in SMB activity.
- Darktrace flags the device successfully logging into a server using administrative credentials via SMBv1, opening the \sect pipe and writing the suspicious and likely malicious file Syssvc.exe to the server’s \temp folder. Immediately after this, Darktrace alerts to the device writing mimikatz.exe to the same folder.

- Figure 2: Darktrace flags the device using Mimikatz.
- Following this step, multiple .tmp and .txt files appear on the target device, indicating that Mimikatz was proceeding to access passwords and hashes.
The novelty of this AI-powered approach is that it does not rely on a string search for the term “mimikatz.exe”; rather, it highlights the unusual behavior that commonly surrounds Mimikatz’ activity. As shown in the screenshot above, this behavior constitutes “New activity”: Darktrace has not seen this type of SMB activity between the source and the destination before. Moreover, SMBv1 is used — highly unusual for the environment. And finally, it is unusual for devices in the target environment to make SMB network drive writes to Temp folders. All these subtle deviations from the customer’s ‘pattern of life,’ taken together, caused Darktrace to alert on the Mimikatz behavior.
Since its introduction to the cyber-threat landscape, Mimikatz has become a highly effective means for cyber-attackers to move laterally inside corporate and government networks. But by empowering security teams to respond before attackers can plunder a network’s entire cache of passwords, AI cyber defenses are thwarting Mimikatz and its copycats alike.