With 90million investigations in 2024 alone, Darktrace Cyber AI Analyst TM is transforming security operations with AI and has added up to 30 Full Time Security Analysts to almost 10,000 security teams.
In today’s high-stakes threat landscape, security teams are overwhelmed — stretched thin by burnout, alert fatigue, and a constant barrage of fast-moving attacks. As traditional tools can’t keep up, many are turning to AI to solve these challenges. But not all AI is created equal, and no single type of AI can perform all the functions necessary to effectively streamline security operations, safeguard your organization and rapidly respond to threats.
Thus, a multi-layered AI approach is critical to enhance threat detection, investigation, and response and augment security teams. By leveraging multiple AI methods, such as machine learning, deep learning, and natural language processing, security systems become more adaptive and resilient, capable of identifying and mitigating complex cyber threats in real time. This comprehensive approach ensures that no single AI method's limitations compromise the overall security posture, providing a robust defense against evolving threats.
As leaders in AI in cybersecurity, Darktrace has been utilizing a multi-layered AI approach for years, strategically combining and layering a range of AI techniques to provide better security outcomes. One key component of this is our Cyber AI Analyst – a sophisticated agentic AI system that avoids the pitfalls of generative AI. This approach ensures expeditious and scalable investigation and analysis, accurate threat detection and rapid automated response, empowering security teams to stay ahead of today's sophisticated cyber threats.
In this blog we will explore:
- What agentic AI is and why security teams are adopting it to deliver a set of critical functions needed in cybersecurity
- How Darktrace’s Cyber AI AnalystTM is a sophisticated agentic AI system that uses a multi-layered AI approach to achieve better security outcomes and enhance SOC analysts
- Introduce two new innovative machine learning models that further augment Cyber AI Analyst’s investigation and evaluation capabilities
The rise of agentic AI
To combat the overwhelming volume of alerts, the shortage of security professionals, and burnout, security teams need AI that can perform complex tasks without human intervention, also known as agentic AI. The ability of these systems to act autonomously can significantly improve efficiency and effectiveness. However, many attempts to implement agentic AI rely on generative AI, which has notable drawbacks.
Broadly speaking, agentic AI refers to artificial intelligence systems that act autonomously as "agents," capable of carrying out complex tasks, making decisions, and interacting with tools or external systems with no or limited human intervention. Unlike traditional AI models that perform predefined tasks, it uses advanced techniques to mimic human decision-making processes, dynamically adapting to new challenges and responding to varied inputs. In a narrower definition, agentic AI often uses generative large language models (LLMs) as its core, using this to plan tasks and interactions with other systems, iteratively feeding its output into its input to accomplish more tasks than are traditionally possible with a single prompt. When described in terms of technology rather than functionality, agentic AI would be deemed as AI using this kind of generative system.
In cybersecurity, agentic AI systems can be used to autonomously monitor traffic, identify unusual patterns or anomalies indicating potential threats, and take action to respond to these possible attacks. For example, they can handle incident response tasks such as isolating affected systems or patching vulnerabilities, and triaging alerts. This reduces the reliance on human analysts for routine tasks, allowing them to focus on high-priority incidents and strategic initiatives, thereby increasing the overall efficiency and effectiveness of the SOC.
Despite their potential, agentic AI systems with a generative AI core have notable limitations. Whether based on widely used foundation models or fully custom proprietary implementations, generative AI often struggles with poor reasoning and can produce incorrect conclusions. These models are prone to "hallucinations," where they generate false information, which can be magnified through iterative processes. Additionally, generative AI systems are particularly susceptible to inheriting biases from training data, leading to incorrect outcomes, and are vulnerable to adversarial attacks, such as prompt injection that manipulates the AI's decision-making process.
Thus, choosing the right agentic AI system is crucial for security teams to ensure accurate threat detection, streamline investigations, and minimize false positives. It's essential to look beyond generative AI-based systems, which can lead to false positives and missed threats, and adopt AI that integrates multiple techniques. By considering AI systems that leverage a variety of advanced methods, organizations can build a more robust and comprehensive security strategy.
Industry’s most experienced agentic AI analyst
First introduced in 2019, Darktrace Cyber AI AnalystTM emerged as a groundbreaking, patented solution in the cybersecurity landscape. As the most experienced AI Analyst deployed to almost 10,000 customers worldwide, Cyber AI Analyst is a sophisticated example of agentic AI, aligning closely with our broad definition. Unlike generative AI-based systems, it uses a multi-layered AI approach - strategically combining and layering various AI techniques, both in parallel and sequentially – to autonomously investigate and triage alerts with speed and precision that outpaces human teams. By utilizing a diverse set of AI methods, including unsupervised machine learning, models trained on expert cyber analysts, and custom security-specific large language models, Cyber AI Analyst mirrors human investigative processes by questioning data, testing hypotheses, and reaching conclusions at machine speed and scale. It integrates data from various sources – including network, cloud, email, OT and even third-party alerts – to identify threats and execute appropriate responses without human input, ensuring accurate and reliable decision-making.
With its ability to learn and adapt using Darktrace's unique understanding of an organization’s environment, Cyber AI Analyst highlights anomalies and passes only the most relevant activity to human users. Every investigation is thoroughly explained with natural language summaries, providing transparent and interpretable AI insights. Unlike generative AI-based agentic systems, Cyber AI Analyst's outputs are based on a comprehensive understanding of the underlying data, avoiding inaccuracies and "hallucinations," thereby dramatically reducing risk of false positives.
90 million investigations. Zero burnout.
Building on six years of innovation since launch, Darktrace's Cyber AI Analyst continues to revolutionize security operations by automating time-consuming tasks and enabling teams to focus on strategic initiatives. In 2024 alone, the sophisticated AI system autonomously conducted 90 million investigations, its analysis and correlation during these investigations resulted in escalating just 3 million incidents for human validation and resulting in fewer than 500,000 incidents deemed critical to the security of the organization. This completely changed the security operations process, providing customers with an ability to investigate every relevant alert as an unprecedented alternative to detection engineering that avoids massive quantities of risk from the traditional approach. Cyber AI Analyst performed the equivalent of 42 million hours of human investigation for relevant security alerts.
The benefits of Cyber AI Analyst will transform security operations as we know it today:
- Autonomously investigates thousands of alerts, distilling them into a few critical incidents — saving security teams thousands of hours and removing risk from current “triage few” processes. [See how the State of Oklahoma gained 2,561 hours of investigation time and eliminated 3,142 alerts in 3 months]
- It decreases critical incident discoverability from hours to minutes, enabling security teams to respond faster to potential threats that will severely impact their organization. Learn how South Coast Water District went from hours to minutes in incident discovery.
- It reduces false positives by 90%, giving security teams confidence in its accuracy and output.
- Delivers the output of up to 30 full-time analysts – without the cost, burnout, or ramp-up time, while elevating existing human security analysts to validation and response
Cyber AI Analyst allows security teams to allocate their resources more effectively, focusing on genuine threats rather than sifting through noise. This not only enhances productivity but also ensures that critical alerts are addressed promptly, minimizing potential damage and improving overall cyber resilience.
Always innovating - Next-generation AI models for cybersecurity
As empowering defenders with AI has never been more critical, Darktrace remains committed to driving innovation that helps our customers proactively reduce risk, strengthen their security posture, and uplift their teams. To further enhance security teams, Darktrace is introducing two next-generation AI models for cybersecurity within Cyber AI Analyst, including:
- Darktrace Incident Graph Evaluation for Security Threats (DIGEST): Using graph neural networks, this model analyzes how attacks progress to predict which threats are likely to escalate — giving your team earlier warnings and sharper prioritization. This means earlier warnings, better prioritization, and fewer surprises during active threats.
- Darktrace Embedding Model for Investigation of Security Threats - Version 2 (DEMIST-2): This new language model is purpose-built for cybersecurity. With deep contextual understanding, it automates critical human-like analysis— like assessing hostnames, file sensitivity, and tracking users across environments. Unlike large general-purpose models, it delivers superior performance with a smaller footprint. Working across all our deployment types, including on-prem and cloud, it can run without internet access, keeping inference local.
Unlike the foundational LLMs that power many generative and agentic systems, these models are purpose-built for cybersecurity, supported by insights of over 200 security analysts and is capable of mimicking how an analyst thinks, to bring AI-based precision and depth of analysis into the SOC. By understanding how attacks evolve and predicting which threats are most likely to escalate, these machine learning models enable Cyber AI AnalystTM to provide earlier detection, sharper prioritization, and faster, more confident decision-making.
Conclusion
Darktrace Cyber AI AnalystTM redefines security operations with proven agentic AI — delivering autonomous investigations and faster response times, while significantly reducing false positives. With powerful new models like DIGEST and DEMIST-2, it empowers security teams to prioritize what matters, cut through noise, and stay ahead of evolving threats — all without additional headcount. As cyber risk grows, Cyber AI Analyst stands out as a force multiplier, driving efficiency, resilience, and confidence in every SOC.
[related-resource]
Additional resources
- Learn more about Darktrace’s unique approach to AI by downloading “The AI Arsenal: Understanding the Tools Shaping Cybersecurity.”
- Connect with Darktrace at RSAC 2025 in San Francisco April 28– May 1st at Booth S-2227 or book a one-on-one meeting with Darktrace experts on-site here.
- Discover the principles guiding Darktrace’s development of responsible AI by downloading “Towards Responsible AI in Cybersecurity.”
Learn more about Cyber AI Analyst
Explore the solution brief, learn how Cyber AI Analyst combines advanced AI techniques to deliver faster, more effective security outcomes
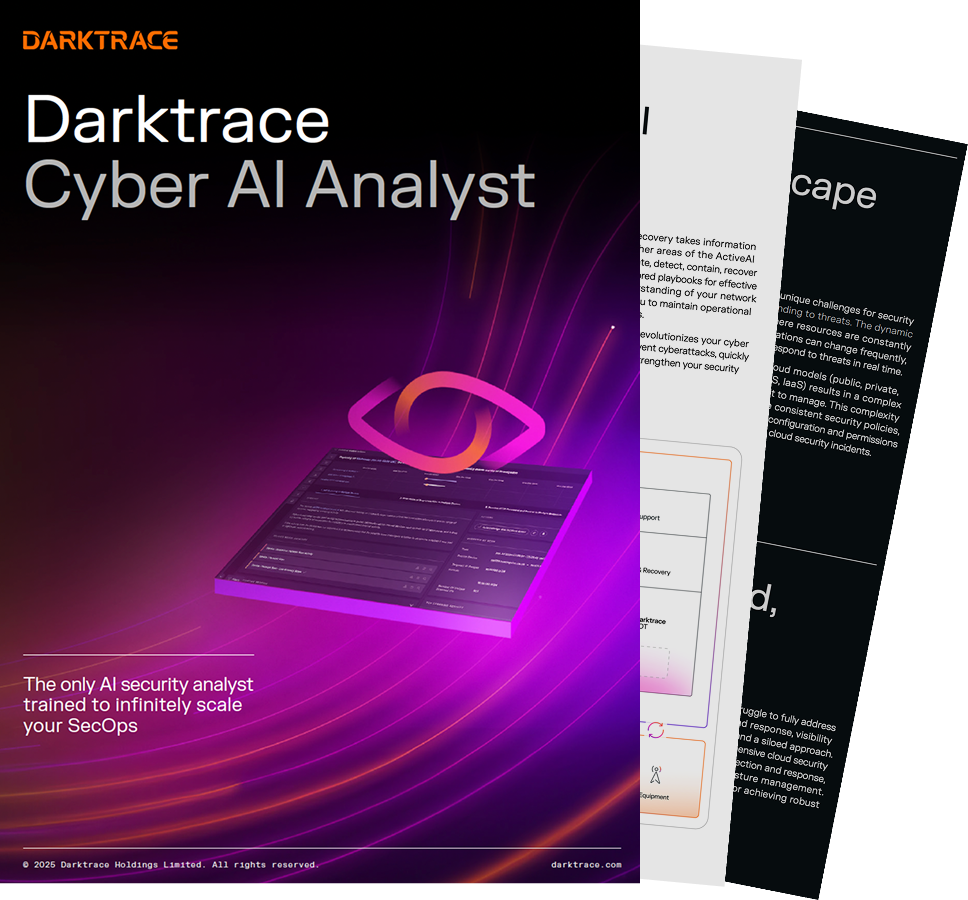